These days there is no shortage of census-type audience data: from set top boxes, from mobile devices, from connected TV’s, from VOD services, from streaming sticks the list goes on and on. This data has the potential to be a valuable source of audience information but has one important limitation in that it typically has metrics based on viewing of households or devices as opposed to the media consumption of individuals. This leaves a question to be answered: How can we estimate the number of individuals reached across that household/device? The answer is Viewers per View factors.
VPVs makes it possible for users to convert household or device impressions to Individual impressions for each viewing instance.
What are Viewers per View factors and why do we need them?
Simply, Viewers per View factors (VPVs) estimate the average number of people viewing per recorded viewing instance. The key reason for VPVs is to allow users to convert household or device level impressions to individual impressions (an instance of viewing by an individual).
Often Viewers Per View factors need to be provided at different levels of granularity (e.g. demographics, device types, genres), recognising key differences across these characteristics in terms of the number of individuals that are likely to be viewing. The greater the granularity of VPVs that may be applied, the more precise the audience profiling of household/device level impressions will be.
How are Viewers per View factors calculated?
Typically, a single source panel is required that will enable the relationship between household/device impressions and the key discriminators to be identified. An example of this is for BARB where the metering of viewing and registering of presence in the room allow the necessary data to be collected. Below is a hypothetical example (eg for a given minute on a small channel):
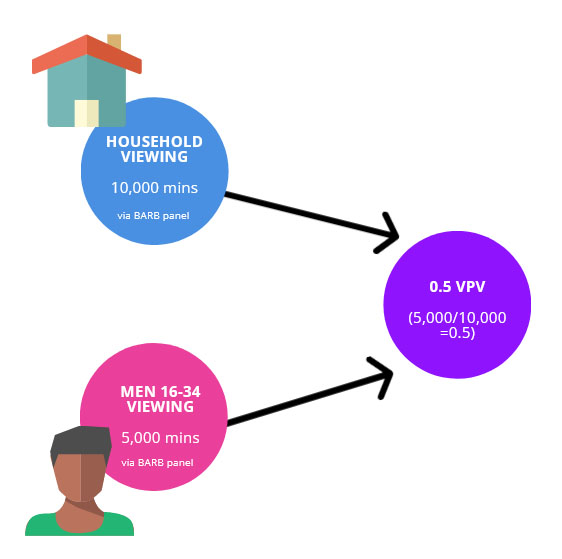
For the example above we have a VPV of 0.5 so we would halve any given number of census impressions to provide an estimate of the number of Men 16-34 impressions.
There may be options to enhance the VPV model further. If census data was available relating to demographics in the household then this additional information could be utilised to provide better estimates. For example, if data was available relating to the composition of households for gender and age, we could calculate a factor related to the number of minutes watched by age 16 to 34 Men within Households containing 16-34 Men. We could then restrict our universe to just households containing Men 16-34 as below and build VPVs from there, as illustrated by the following:

What are Login factors?
Another metric used to estimate the numbers of Viewers Per View are Login Factors. Login factors work identically to VPVs however are used in a scenario where a broadcaster has information on one of the viewers, the “logged in” viewer (hence “Login” factors). Users of these VPVs will be able to estimate the viewing of the “logged in” viewer as well as the other co-viewing groups. As an example, we have viewing of live Sports on a TV set over a certain period where Men 16-34 have been the “logged in” viewer. For Men 16-34, a VPV factor can be calculated from the single source panel which allows us to calculate the number of individual minutes viewed where Men 16-34 have viewed with Women 16-34; Children 4+ and so on. Below is an example of a set of Login factors for Men 16-34 showing the factors for Men 16-34 co-viewing with any of the demographic groups. The "Final login factor” in the diagram is the sum of the demographic based factors (where the combination of the demographic based factors make up the overall universe).

This means that, when a 16 to 34 year old male is watching live sports on a TV set, the average number of viewers is 1.27. Therefore if the data tells us we have 100,000 instances of live sports being watched via a TV set, where men 16 to 34 are the “logged in” viewer, the total individual impressions is 100,000*1.27 = 127,000.
Discrimination within VPVs
An important part of VPV production is that they precisely estimate the number of viewers per view within given devices, genres, days etc. Some of these variables are large discriminators of VPVs and some have less of an impact. Taking devices as an example, a smartphone VPV is always going to be 1 or very close to 1 as it is a personal device where co-viewing is extremely improbable. Comparing this to VPVs for set-top boxes or TVs, these are likely to be considerably larger factors. Similarly with time of the day, a VPV representing 8pm is likely to be larger than a VPV representing 4am since co-viewing is more likely in the evening. The following diagram represents this discrimination for a variety of variables:

How do we ensure the reliability of Viewers Per View/Login Factors?
VPVs need to account for as many of the characteristics available for that session (e.g. device, time, genre) and the reporting requirements (i.e. demographics). As such, a detailed interlaced matrix of VPVs is required and single source data cannot directly provide a measure for all levels of fragmentation. To ensure the factors used to estimate co-viewing are reliable, RSMB will on occasion implement a collapsing routine when using the panel data to calculate factors. This means that if one factor has come from a very small sample or an unreliable sample, it will be combined with another factor to create a more reliable Viewers per View estimate. In order to know when a collapsing routine should be applied to a VPV, RSMB use statistical criteria based on sample size and variation within the sample and dictate cut off points. Where this criteria is not met, the applicable VPV will be collapsed to a more robust equivalent until this criteria is met. The key thing here is there should be a robust and reliable set of co-viewing factors following the collapsing routine, however the VPVs should not lose too much of their granularity and should still retain the most import discriminators as far as possible. To retain this granularity, RSMB have developed a bespoke “Decision Tree”. This gives an order to the collapsing routines ensuring that variables which heavily affect the VPVs themselves are left to the end. These “Decision Trees” are built following in-depth and structured analysis by RSMB.
What does the future hold for Viewers Per View Factors?
With Viewers Per View factors finding their way into many aspects of RSMB’s industry work (e.g, Broadcaster CFlight and BARB’s Advanced Campaign Hub) there is an increased importance on the provision of reliable accurate factors. RSMB continues to develop and enhance our methodologies for VPVs to ensure we deliver the best possible solutions for our customers.